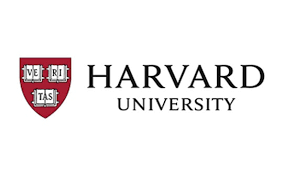
About this course
Are you ready to scale your (tiny) machine learning application? Do you have the infrastructure in place to grow? Do you know what resources you need to take your product from a proof-of-concept algorithm on a device to a substantial business?
Machine Learning (ML) is more than just technology and an algorithm; it's about deployment, consistent feedback, and optimization.
MLOps is a systematic way of approaching Machine Learning from a business perspective.
This course will teach you to consider the operational concerns around Machine Learning deployment, such as automating the deployment and maintenance of a (tiny) Machine Learning application at scale. In addition, you’ll learn about relevant advanced concepts including neural architecture search, allowing you to optimize your models' architectures automatically; federated learning, allowing your devices to learn from each other; and benchmarking, enabling you to performance test your hardware before pushing the models into production.
Are you ready for a billion users?
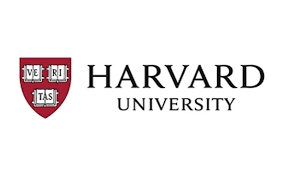
About this course
What do you know about TinyML? Tiny Machine Learning (TinyML) is one of the fastest-growing areas of Deep Learning and is rapidly becoming more accessible. This course provides a foundation for you to understand this emerging field.
TinyML is at the intersection of embedded Machine Learning (ML) applications, algorithms, hardware, and software. TinyML differs from mainstream machine learning (e.g., server and cloud) in that it requires not only software expertise, but also embedded-hardware expertise.
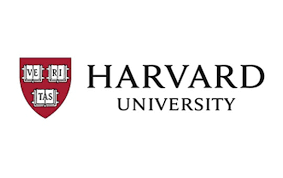
About this course
Do you know what happens when you say “OK Google” to a Google device? Is your Google Home always listening?
Following on the Foundations of Tiny ML course, Applications of TinyML will give you the opportunity to see tiny machine learning applications in practice.
This course features real-world case studies, guided by industry leaders, that examine deployment challenges on tiny or deeply embedded devices.
Tiny Machine Learning (TinyML) is one of the fastest-growing areas of deep learning and is rapidly becoming more accessible. The second course in the TinyML Professional Certificate program, Applications of TinyML shows you the code behind some of the world’s most widely-used TinyML devices.
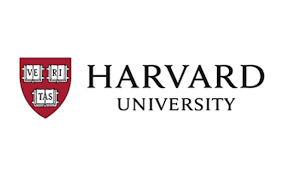
About this course
Have you wanted to build a TinyML device? In Deploying TinyML, you will learn the software, write the code, and deploy the model to your own tiny microcontroller-based device. Before you know it, you’ll be implementing an entire TinyML application.
A one-of-a-kind course, Deploying TinyML is a mix of computer science and electrical engineering. Gain hands-on experience with embedded systems, machine learning training, and machine learning deployment using TensorFlow Lite for Microcontrollers, to make your own microcontroller operational for implementing applications such as voice recognition, sound detection, and gesture detection.
The course features projects based on a TinyML Program Kit that includes an Arduino board with onboard sensors and an ARM Cortex-M4 microcontroller.